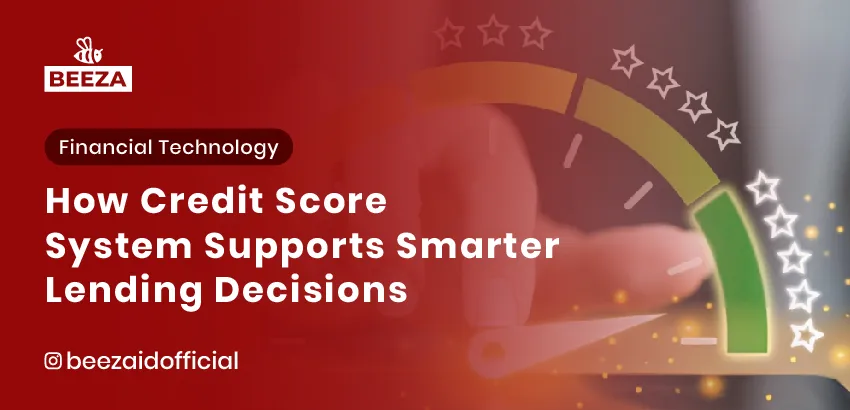
In the modern financial world, credit score systems have become essential for financial institutions facing challenges in making quick and accurate lending decisions. Manual processes often lead to inaccuracies and delays in decision-making. This is where technology-based credit scoring systems play a critical role in accelerating and enhancing the process, providing financial institutions with a more efficient tool for assessing creditworthiness and mitigating risk.
Advantages of Technology-Based Credit Scoring Systems
Technology-based credit scoring systems offer several advantages over traditional methods. Firstly, they utilize vast amounts of data from various sources, including payment histories, social media activities, and even non-traditional metrics like utility payments. This comprehensive data collection enables a more nuanced understanding of a borrower’s financial behavior, leading to more accurate risk assessments. Secondly, the automation of the credit scoring process significantly reduces the time taken for loan approvals, allowing institutions to serve customers more efficiently and stay competitive in a fast-paced market. Lastly, these systems often incorporate machine learning algorithms, which continuously improve over time as they process more data, enhancing predictive accuracy and helping institutions make more informed lending decisions.
The Role of Credit Score Systems in Lending Decisions
Technology-based credit score systems play a crucial role in improving lending decisions. By leveraging integrated data and in-depth analysis, financial institutions can enhance the accuracy of their lending decisions. Here are some of the key benefits:
- Higher Accuracy: Modern credit score systems integrate various data sources, providing more accurate assessments of a borrower’s creditworthiness.
- Streamlined Process: Automation in credit evaluation allows the loan approval process to be faster and more cost-effective.
- Better Risk Management: With advanced technology, financial institutions can better assess and manage risks, reducing the likelihood of defaulting loans.
Features and Value of Technology-Based Credit Scoring Systems
Technology-based credit scoring systems offer several features that provide added value to financial institutions, including:
- Comprehensive Data Integration: This system integrates data from various sources, including financial transactions, demographic information, and credit history. This allows for a more holistic assessment of a borrower.
- Guaranteed Security and Compliance: With high security standards and compliance with applicable regulations, this system ensures the safety of customer data.
- Machine Learning (ML): It uses machine learning to provide more accurate predictions of creditworthiness, adapting to new patterns that may not be identified by traditional analyses.
- Real-Time Decisioning: Real-time data processing enables faster loan decisions, improving the customer experience.
- Scalability and Flexibility: The system is designed to be scalable, allowing it to meet the growing needs of financial institutions over time.
One example of implementing this system can be found in the solutions offered by technology companies, which have leading products in data management and credit analysis, enabling more efficient and accurate decision-making.
Case Study: The Impact of Credit Score Systems on Lending Decisions
Problem Introduction:
A large financial institution was facing issues with a manual loan evaluation process that was time-consuming and unreliable. This led to increased default rates and affected the customer experience.
Problem Details:
- The long loan approval process reduced service speed.
- Inaccurate credit assessments resulted in higher-risk loans.
- The lack of data integration made managing data more complex and prone to errors.
Technology Solution:
To address these issues, the financial institution decided to implement a technology-based credit scoring system, which provided automated data integration and machine learning-based analysis. The solution included:
- Automated Credit Evaluation Process: The credit scoring system automated the evaluation process, reducing approval time and minimizing human error.
- Multi-Source Data Integration: Combining data from various sources, including financial data, transaction information, and credit history, for a more comprehensive analysis.
- Machine Learning-Based Analysis: The system used machine learning algorithms to assess creditworthiness more accurately, helping identify risks more effectively.
Outcome:
- Loan approval times were reduced by 50%.
- Defaulted loans decreased by 20% due to more accurate credit assessments.
- Customer satisfaction improved with faster and more transparent processes.
Conclusion
The implementation of technology-based credit scoring systems offers numerous advantages for financial institutions in making better lending decisions. By improving accuracy, efficiency, and risk management, financial institutions can offer better and faster services to their customers. This technology is a key driver in creating smarter and more informed lending decisions.